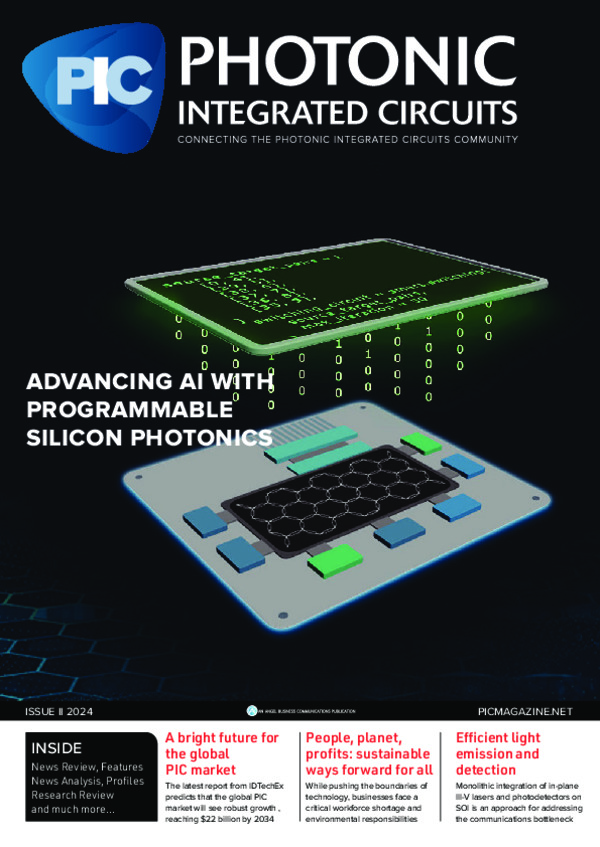
Advancing AI with programmable silicon photonics
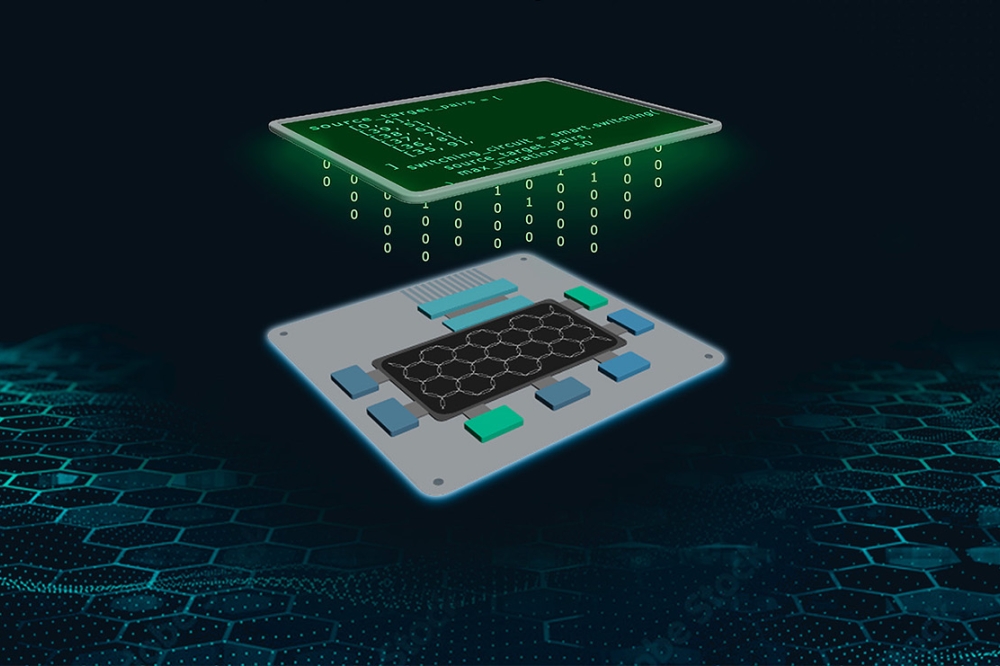
As traditional AI infrastructure approaches its limits, optical circuit switching offers a promising route to higher performance. iPronics’ software-defined silicon photonics engine can deliver the low latency, high speed, and flexibility to support the next generation of AI clusters.
By Ana González, vice president of business development, iPronics
The AI revolution unfolding today promises to open new frontiers in wide-ranging fields, from designing more targeted drugs in medicine to achieving real-time augmented reality. These exciting machine learning (ML) applications have highlighted the importance of large-scale compute capabilities, and the growing demand for powerful ML models has driven an increase in the size of deep neural networks, as well as the datasets used to train them.
As this technology reshapes our world, it is having a profound impact on the landscape of the datacentre industry, demanding more robust, efficient, and scalable solutions. Training large language models (LLMs) involves complex and power-intensive computational tasks, with higher requirements of network bandwidth, latency, and advanced network management systems. All of this creates a pressing need for entirely new ways to build interconnects for distributed ML systems. When AI leaders were recently asked about the key considerations for expanding their AI compute infrastructure in 2024, they reported that flexibility and speed are the top drivers, with 65 percent and 55 percent of respondents citing these factors, respectively. Optimising GPU utilisation is another major concern for 2024-2025, with the majority of GPUs currently underutilised during peak times [1].
As the demands of AI compute infrastructure grow, traditional electronic solutions are reaching their limits in terms of flexibility and rapid scaling. To take AI to the next level, the field of photonics offers a promising path forward, with optical circuit switching (OCS) emerging as a key technology for dynamic network reconfiguration and efficient data flow.
In this article, we present the limitations of current infrastructure for AI clusters, and how OCS technology – particularly software-defined silicon photonics engines – can overcome them.
Figure 1. Sketch of the chip under development at iPronics for AI clusters and datacentre applications.
Fibre links and network topologies
Hyperscale datacentres usually use a folded Clos topology, also called a spine-leaf architecture. In this configuration, servers in the rack are connected to the top-of-rack (ToR) switches with short copper cables that are 1-2 m long and carry 25G or 50G signalling. The ToR is then connected through a fibre cable to a leaf switch at the end of the row or in another room. All the leaf switches in a datacentre connect to all the spine switches.
AI clusters, on the other hand, require much more connectivity between GPU servers, but have fewer servers per rack (due to heat constraints). Each GPU server is connected to a switch within the row or room. These links require 100G to 400G over distances that cannot be supported by copper.
NVIDIA’s GPU server DGX H100 has 4 x 800G ports to switches (operated as 8 x 400GE), 4 x 400GE ports to storage, and 1GE and 10GE ports for management. A DGX SuperPOD can contain 32 of these GPU servers connected to 18 switches in a single row. This represents a remarkable increase in the number of fibre links in the data hall, indicating a potential need for alternative network topologies and technologies that can overcome the scaling challenge.
Optical circuit switching (OCS) technologies offer benefits compared with electronic packet switches (EPS), including low latency, energy efficiency, and data rate and wavelength agnosticism. OCS is agnostic to bit rate, protocol, framing, line coding, and modulation formats. Leveraging OCS is equivalent to physical re-cabling of the fibre links, without requiring any actual re-cabling, to adapt the system configuration to match workload placement.
Among the main benefits of OCS technology in datacentres is its flexibility, since it can support different network topologies that can adapt to the communication patterns of individual services. Additionally, optical switching can replace subsets of electronic switches within static topologies, reducing power, latency, and cost. Moreover, the cost of OCS systems does not grow with increasing data rates.
Figure 2. New network infrastructure for AI clusters, called rail-only,
where the spine switches are removed and all the uplinks connecting rail
switches to the spine are repurposed as down-links to GPUs.
Google has been the first hyperscaler to deploy OCS on a large scale for datacentre networking; by employing circulators to realise bidirectional links through the OCS, the company has effectively doubled the OCS radix [2]. Google implemented a datacentre interconnection layer employing micro-electro-mechanical systems (MEMs)-based OCS to enable dynamic topology reconfiguration, centralised software-defined networking (SDN) control for traffic engineering, and automated network operations for incremental capacity delivery and topology engineering [3].
In AI clusters, OCS technology can also be highly valuable for bandwidth-intensive and latency-sensitive applications such as ML training. Optical switches that are commercially available today have a reconfiguration latency of around 10 ms, which makes them suitable for circuits that last through the entire training process.
Flexibility for network adaptations
In most datacentres, there are two levels of network adaptations that can save capex and opex: traffic engineering and topology engineering. These two levels operate on distinct timescales.
The first level, traffic engineering, operates on top of the logical topology, and aims to optimise traffic forwarding across different paths, based on the real-time demand matrix representing communication patterns. Traffic engineering is the inner control loop that responds to topology and traffic changes at a granularity of seconds to minutes, depending on the urgency of the change. In this context, OCS technology allows for traffic-aware topology that can accommodate a particular load and avoid indirect paths.
The second level, topology engineering, adapts the topology itself to increase bandwidth efficiency. In a datacentre, the varying demand matrix needs to be satisfied, while also leaving enough headroom to accommodate traffic bursts, network failures, and maintenance. In datacentres, generating short-term traffic predictions is challenging, so topology engineering is much slower than traffic engineering.
However, large-scale ML requires more frequent topology reconfiguration, with its high bandwidth requirements and relative predictability of traffic patterns, which shift intensive communication among changing subsets of computation and accelerator nodes. Large-scale AI clusters simultaneously manage multiple jobs with varying communication needs.
If a job requires a large amount of inter-group traffic, unrestricted routing can allow data to travel freely across the network. However, it also introduces competition between unrelated jobs, causing unpredictable performance. Furthermore, indirect routing consumes more capacity and increases round-trip time, impacting flow completion time, particularly for small flows.
Figure 3. OCS replacing spine switches, providing high bandwidth and
reconfigurability in connecting high-bandwidth domains in different
racks.
OCS technology can address this challenge in AI clusters by leveraging topology engineering. In particular, optical switching can be used to effectively “re-cable” all the links of the groups at job launch time, to provide increased bandwidth between the groups involved in that job.
This higher bandwidth reduces the need for indirect routing, thereby potentially reducing latency and increasing delivered end-to-end bandwidth. OCS can also eliminate all network interference between unrelated jobs, while supporting a range of novel network topologies, meaning that the topology can be tailored to suit the communication patterns of individual services [4].
Reducing transmission latency
Some of the most exciting emerging ML applications include mobile Augmented Reality (mobile AR) and Internet of Things (IoT) analytics. However, a major challenge of realising these technologies is that data needs to be processed with tight latency constraints.
Network latency during data communication comprises two components: static latency and dynamic latency. Static latency refers to delays associated with data serialisation, device forwarding, and electro-optical transmission. It is determined by the capabilities of the forwarding chip and transmission distance, and has a constant value when the network topology and communication data volume are fixed. Conversely, dynamic latency encompasses delays due to queuing within switches and due to packet loss and retransmission, often caused by network congestion. This component can significantly affect network performance.
Addressing these problems is critical for latency-sensitive ML applications, and OCS technology can eliminate some sources of delay. For instance, optical switches do not entail any per-packet processing, as they allow a shift from traditional optical-electrical-optical (OEO) to all-optical (OOO) interconnects. Latency is therefore set by the speed-of-light propagation delay – 5 ns/m in optical fibre, and 3.3 ns/m in free space. By comparison, an equivalent EPS would add on the order of 10-100 ns of delay per network hop. Additionally, as mentioned above, OCS-enabled topology engineering can rewire fibre links to avoid the use of indirect, higher-latency paths.
Overcoming challenges with software-defined silicon photonics
In light of the challenges outlined above, software-defined photonics emerges as a transformative solution, especially in the context of AI infrastructures. The technology provides greater benefits than MEMs-based optical switching, such as faster reconfiguration time.
Current OCS equipment is limited to coarse-grained reconfigurations, because the switching times exceed milliseconds, limiting its applicability to relatively long-lived applications such as backups and virtual machine migration. This might represent an opportunity for faster switching speed and/or smaller radix, and lower-cost OCS in lower layers of the datacentre network for shorter or more bursty traffic flows (i.e. ToR to AB traffic) or flexible bandwidth provisioning.
Figure 1 shows a sketch of a software-defined silicon photonics chip that iPronics is developing for interconnect applications. This chip is based on programmable silicon photonics technology [5] and consists of tuneable elements in a mesh architecture that can be controlled by software. Other building blocks can be incorporated to add functionalities including monitoring photodetectors and matrix multiplication.
The system contains a software framework and control system that includes an electronic processing unit (PU), multi-channel electronic driver array (MEDA), and multi-channel electronic monitor array (MEMA) for precise actuation and real-time optimisation.
To ensure seamless integration and flexibility, the software layer incorporates application programming interfaces (APIs), enabling interaction between the software and its underlying photonic hardware components. This allows various levels of user interaction, from manual adjustments to fully autonomous configurations, such as integration with software-defined networking (SDN) for advanced network management, optimisation, and automation.
Future AI clusters for heavy ML workloads
The rise of network-heavy ML workloads has led to the dominance of GPU-centric clusters that typically employ two types of connections: (i) a few GPUs residing within a high-bandwidth (HB) domain through a short-range interconnection, and (ii) any-to-any GPU communication using remote direct memory access (RDMA)-enabled network interface cards (NICs) connected in a variant of a Clos network.
However, recent research has demonstrated that network cost can be reduced by up to 75 percent by following a “rail-only” connection. In this new architecture, outlined in Figure 2, LLM training traffic does not require any-to-any connectivity across all GPUs in the network. Instead, it only requires high-bandwidth any-to-any connectivity within small subsets of GPUs, and each subset fits within a HB domain [6].
We therefore suggest using reconfigurable optical switches to provide greater flexibility for interconnections across HB domains even in different racks (see Figure 3). Such a design also allows for the reconfiguration of some connections across rails for forthcoming workloads that behave differently from LLMs. Previous research has shown that µs reconfiguration latency is close to optimal for ML [7]. Software-defined silicon photonics engines offer a µs reconfiguration time, making them particularly suitable for use in optical interconnects for distributed ML training clusters. Building on this, it seems that fast reconfigurable switches are going to be essential in elastic scenarios where the cluster is shared across multiple jobs, with servers joining and leaving different jobs unexpectedly, or when large, high-degree communication dominates the workload.
Our vision at iPronics is that software-defined silicon photonics engines will play a key role in evolving the next generation of AI clusters to optimise dynamic traffic engineering and topology engineering. Offering low transmission latency and a µs reconfiguration time, this OCS technology can fulfil the requirements of new, more cost-efficient AI infrastructures for reconfigurable and flexible optical switches to connect HB domains that can be in distant racks. As AI increasingly reshapes our world, software-defined photonics can support it in realising its transformative potential.